원래는 논문리뷰를 하려다가 갑자기 틀어 정리 글을 쓰게 되었다. 허허헣
이런 글을 처음 쓸려니까 너무 어려운거 같다..ㅋㅋ
정리를 좀 더 체계적으로 할 필요성을 느낀다ㅏ다ㅏㅏ
Abstract
Past :
The sequnce transduction model
- include complex recurrent or convolutional neural network
- encoder and decoder
- BEST : connected the encoder and decoder through attention mechanism
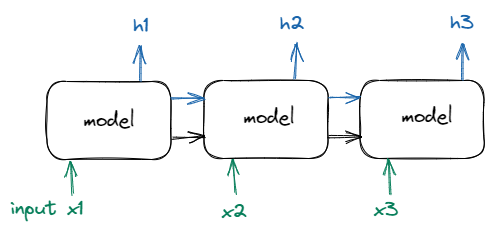
- 병렬화 어려움
- sequence 길이가 길수록 critical한 메모리 제약 문제
- 장기 의존성 문제(Long-Term Dependency)
This Paper :
Transformer : only use attention mechanism dispending with reurrence and convolutions
- parallelizable
- less train time
- machine translation / Constituency Parsing
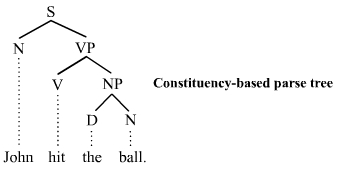
- parsing : 문장에서 단어들이 어떤 구조로 엮여 있는지 나타내는 것
- constituency parsing : 문장이 구 단위로 묶여가면서 구조를 이뤄가는 방법
(어순이 고정적인 언어(영어)에서 사용)
Model Architecture
Hyper Parameter in Paper
- $d_{model}=512$
- $N = 6$
- $num \; of \; head = 8$
- $d_{ff} = 20$(feed forward 의 hidden layer 크기)
Encoder
- N=6, 동일한 layer를 쌓아 구성
- Multi-Head Attention
- position-wise Fully Connected feed forward
- 각 Sub-Layer는 Residual Connection 및 Layer Nomalization 적용 ➡ ${LayerNorm(x + SubLayer(x))}$
Residual Connection 적용을 용이하게 하기 위해, Sub-layer, Embedding, Output Dimension을 512로 통일
( ∵ Residual Connection 적용을 위해선, Input과 연결된 Output의 Dimenstion이 동일해야 함)
- 각 Encoder Layer는 이전 Layer로부터 입력의 모든 위치를 포함한 정보를 활용
Decoder
- N=6, 동일한 layer를 쌓아 구성
- Masked Multi-Head Attention
- 어떤 i번째 위치에서 prediction을 진행할 때, 미래의 위치들에 접근하는 것을 불가능하게 하고 해당 위치 i와 그 이전의 위치들에 대해서만 의존하도록 masking 기법을 이용
- Encoder의 Output에 대해 Multi-Head Attention
- position-wise Fully Connected feed forward
- Query Key Value
- Query : 이전 Decoder Layer에서
- Key 와 Value : encoder에서의 output에서
- Decoder에서 모든 position 정보 사용 방지 ➡ auto-regressive property를 위해(정보 흐름 방지)
Self-Attention
1) 입력 벡터(여기서는 Word Embedding)를 활용하여, 3개(Query, Key, Value)의 백터를 생성
2) Score 계산 : Query와 Key 백터를 내적
3) Key 백터 크기의 제곱근만큼을 Scaling ➡ 더 안정적인 gradient를 위해
4) Softmax 계산을 통해, 전체 Score를 정규화(전체 합 1)
5) Value 백터에 Softmax값을 곱함 (중요도 판단)
6) Value 백터를 가중치 합 계산
Multi-Head
- Multi-Head 개념을 추가하여, Attention Layer의 성능을 향상
- 단일 Attention은 Averaging 효과로 다양한 Attention 탐지가 억제됨
- Head의 축소된 차원(${d_{v} × Head=64 × 8=512}$)으로 인해,
총 계산 비용은 Single-Head Attention($d_{model}=512$)과 유사
자세한 내용 https://kmhana.tistory.com/28 참고 하기
• Attention을 병렬 수행하여, $d_{v}$ − dimensional Output 값을 생성
1) 다른 위치에 있는 단어를 집중(Attention)하는 능력 향상
- 예를 들어, 'It'이 가리키는 단어를 알고 싶을 때 유용
2) 여러 "Representation Sub-space"를 제공
- 8개의 Scaled Dot-Product Attention을 사용하여, 여러 표현의 Attention을 학습 가능토록 함
- ①서로 다른 위치에 있는 ②서로 다른 Represntation Sub-space의 정보를 ③결합하여, Attention 할 수 있음
Position Encoding
- i : 임베딩 벡터의 위치
- pos : Word(token)의 위치
연구자들이 sin, cos 기반 encoding / 학습 가능한 위치 임베딩 사용 ➡ 두 방식 거의 동일한 결과
결국, sinusoidal version 선택
➡ 모델을 훈련할 때 사용한 길이보다 더 긴 sequnce에 대해 얼마든지 extrapolate 할 수 있기 때문
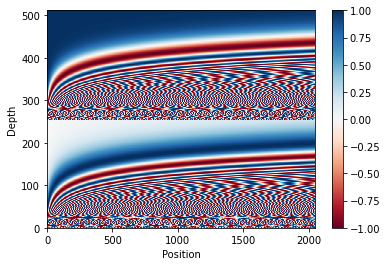
- Input Embedding + Positional Encoding ➡ input
- Sequence 정보를 모델링하는데, 한계가 있을 수 있음 ➡ 상대/절대 적인 token의 위치를 전달해주기 위해서
- 각 단어의 위치나, 단어 사이의 거리를 결정하는데 도움을 줌
Result
REFERENCE
https://velog.io/@changdaeoh/Transformer-%EB%85%BC%EB%AC%B8%EB%A6%AC%EB%B7%B0
'Paper Review > NLP' 카테고리의 다른 글
ELECTRA: Pre-training Text Encoders as Discriminators Rather Than Generators 정리 리뷰 (1) _ intro, method (0) | 2022.07.09 |
---|
댓글